REGRESSION ANALYSIS: MEANING
The statistical technique of estimating or predicting the unknown value of a dependent variable from the known value of an independent variable is called regression analysis. Sir Francis Galton introduced the concept of regression for the first time in 1877 where he studied the case of one thousand fathers and sons and concluded that the tall father tends to have tall sons and short fathers have short sons, but the average height of the sons of a group of tall fathers is less than that of the fathers and the average height of the sons of a group of short fathers is greater than that of the fathers.
ACCORDING TO MORRIS M. BLAIR
“Regression is the measure of the average relationship between two or more variables in terms of the original units of the data.”
ACCORDING TO YA LUN CHOU
“Regression analysis attempts to establish the nature of relationship between variables….and thereby provide a mechanism for prediction or forecasting.”
TYPES OF REGRESSION ANALYSIS
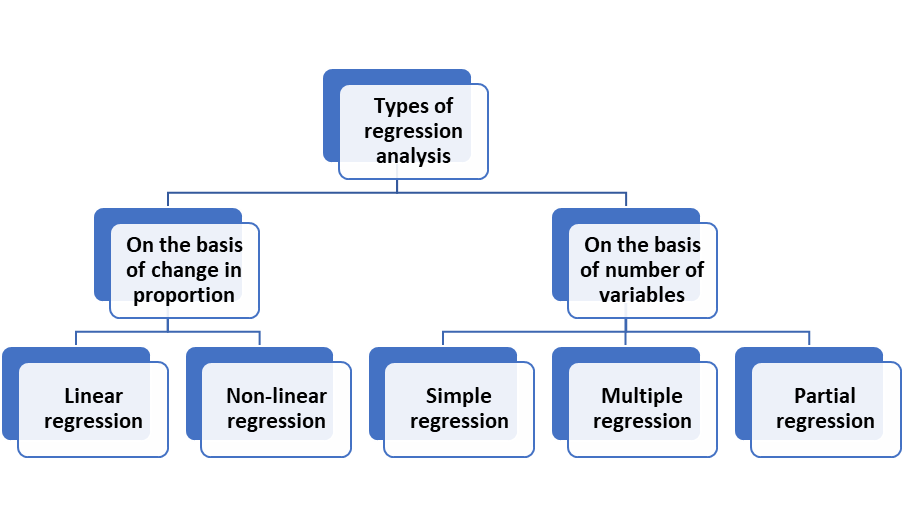
BASIS OF CHANGE IN PROPORTIONS
On the basis of proportions, the regression can be classified into the following categories:
1. Linear regression and
2. Non-linear regression.
LINEAR REGRESSION ANALYSIS
When dependent variable moves in a fixed proportion of the unit movement a independent variable, it is called a linear regression. Linear regression, when plotted on graph paper, forms a straight line. Mathematically the relation between X and Y variables a be expressed by a simple linear regression equation as under
yi= a+bxi+ei
Where a and b are known as regression parameters, ei denotes residual terms, xi presents value of independent variable and yi is the value of dependent variable. ‘a’ expresses intercept of the regression line of y on x. i.e. value of dependent variable say y, when the value of independent variable, that is ‘x’ is zero. Again ‘b’ denotes the slope of regression line of y on x. Again, ei denotes the combined effect of all other variables, (not taken in the model) on y. This equation is known as classical simple linear regression model.
NON-LINEAR REGRESSION ANALYSIS
Contrary to the linear regression model, in non-linear regression, the value of dependent on variable say ‘y’ does not change by a constant absolute amount for unit change in the value of the independent variable, say ‘x’. If the data are plotted on a graph, it would form a curve rather than a straight line. This is also called curvi-linear regression.
ON THE BASIS OF NUMBER OF VARIABLES
On the basis of number of variables regression analysis can be classified as under:
1. Simple Regression.
2. Partial Regression.
3. Multiple Regression.
SIMPLE REGRESSION
When only two variables are studied to find the regression relationships, it is known simple regression analysis. Of these variables, one is treated as an independent variable while the other as dependent one. Functional relationship between the price and the demand may be noted as an example of simple regression.
PARTIAL REGRESSION
When more than two variables are studied in a functional relationship but the relationship of only two variables is analysed at a time, keeping other variables as constant, such a regression analysis is called partial regression.
MULTIPLE REGRESSION
When more than two variables are studied and their relationships are simultaneously worked out, it is a case of multiple regression.
Study of the growth in the production of wheat in relation to fertilizers, hybrid seeds, irrigation etc., is an example of multiple regression.
REGRESSION LINES
A regression line is a graphic technique to show the functional relationship between the two variables X and Y i.e. dependent and independent variables. It is a line which shows average relationship between two variables X and Y. Thus, this is a line of average. This is also called an estimating line as it gives the average estimated value of dependent variable (Y) for any given value of independent variable (X).
ACCORDING TO GALTON
“The regression lines show the average relationship between two variables”
ACCORDING TO J.R. STOCKTON
“The device used for estimating the value of one variable from the value of the other consists of a line through the points, drawn in such a manner as to the average relationship the two variables. Such a line is called the line of regression.”
PROPERTIES OF REGRESSION ANALYSIS
1. Both the regression coefficients bxy and byx cannot be greater than unity, i.e. either both are less than unity or one of them must be less than unity. In other words, the square root of the product of two regression coefficient must be less than or equal to 1 or -1 or
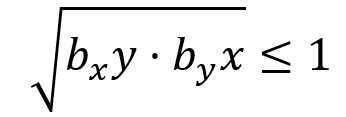
2. Both the regression coefficients will have the same sign i.e.
a. If bxy is positive, then byx will also be positive.
b. If bxy is negative, then byx will also be negative.
c. Both bxy and byx must have ssame signs. If both are positive, r will be positive and vice-versa.
d.
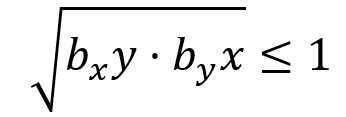
3. Correlation coefficient is the geometric mean between regression coefficients i.e.
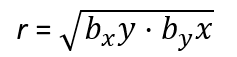
4. The arithmetic mean of bxy and byx is greater than or equal to coefficient of correlation i.e.
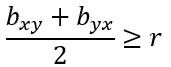
5.

r= bxy= byx
6. If r= 0 then bxy=byx both are zero.
7. If bxy= byx then it is equal to coefficient of correlation. It means r= bxy= byx
8. Regression coefficients are independent of change of origin but not of scale.
USES OF REGRESSION ANALYSIS IN BUSINESS
The technique of regression is considered to be the most useful statistical tool applied in various fields of sociological and scientific disciplines. It is helpful in making quantitative predictions in business in the behaviour of the related variables. Following are some of the main uses of regression analysis in business.
1. Prediction of Unknown Value: The regression analysis technique is very useful in predicting the probable value of an unknown variable in response to some known related variable. For example, the estimate of demand on a given price can be made if the demand and given price are functionally related to each other.
2. Nature of Relationship: The regression device is useful in establishing the nature of the relationship between two variables.
3. Estimation of Relationship: Regression analysis is extensively used for the measurement and estimation of the relationship among variables. It is an important statistical device which provides basis for analysis and interpretation in research studies.
4. Calculation of Co-efficient of determination: The regression analysis provides regression co-efficients which are generally used in calculation of Co-efficient of Correlation. The square of co-efficient of correlation (r) is called the coefficient of determination which measures the degree of association that exists between two variables. The higher the value of r2 the better are regression lines and more useful are the regression equations for prediction and estimation.
5. Helpful in calculation of error: Regression analysis is very helpful in estimating the error involved in using the regression line as a basis for estimation.
6. Policy formulation: The predictions made on the basis of estimated inter-relationship through the techniques of regression analysis provide sound basis for policy formulation in socio- economic fields.
7. Touch stone of hypothesis: The regression tool is considered to be a pertinent testing tool in statistical methodology. It is used in testing the laws and theories of the social sciences as well as natural sciences where the interrelationship between the variables is involved.
LIMITATIONS OF REGRESSION ANALYSIS
Despite all utilities, the regression analysis, too, has various limitations. The following are some of the limitations of regression analysis
1. Assumption of linear relationship: Regression analysis is based on the assumption that there always exists linear relationship between related variables. The linear type of relationship does not always exist in the field of social sciences. In these fields non-linear or curvilinear relationships are most commonly found.
2. Assumption of static condition: While calculating the regression equations a static condition of relationship between the variables is presumed. It is supposed that the relationship has not changed since the regression equation was computed. Such type of assumption has made the regression analysis a static one and hence reduces its applicability in social fields.
3. Study of relationship in prescribed limits: The linear relationship between the variables can only be ascertained within limits. When prescribed limits are crossed, the results become incorrect or inconsistent Such a relation exists between price and profits. When price is higher the profits are high to a certain limit. When the prices are abnormally high the profits may decline due to entry of new firms increasing thereby the supply of the commodity. Despite all shortcomings and limitations the regression technique is considered to be a most useful statistical device.