MEASURES OF DISPERSION IN STATISTICS
STANDARD DEVIATION
The concept of standard deviation was introduced by Karl Pearson in 1893.
Standard deviation is “the square root of the mean of the deviations squared, or the root mean square deviation from the mean”. Standard deviation is denoted by the Greek letter sigma (σ).

Instead of ignoring the signs as in the calculation of mean deviation, we can make them all positive by squaring them. But by the squaring operation, the deviations from the mean will not give a zero sum but a positive number, and each deviation will contribute to the sum of squares regardless of sign. Then in order to compensate for squaring the deviations the square root is taken.
The standard deviation is calculated as follows:
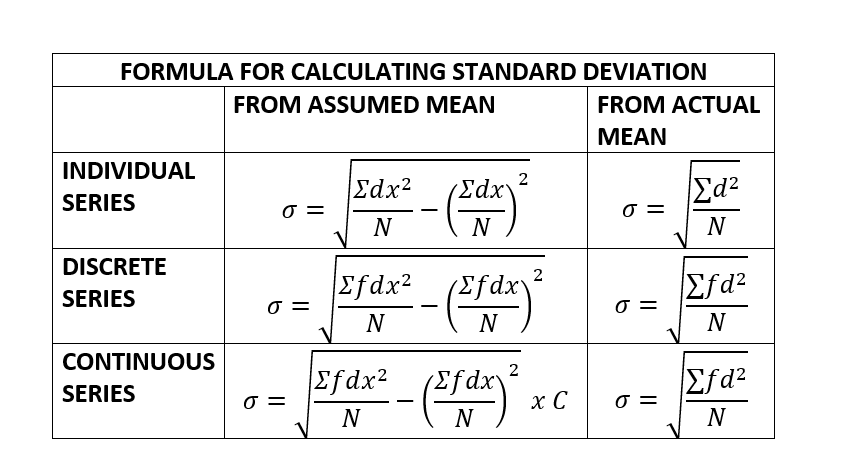
PROPERTIES OF STANDARD DEVIATION
- Standard deviation is independent of change of origin. In other words, if any constant “K” is added or subtracted from all the values of variable ‘X’ it does not affect the standard deviation. Mathematically,
S.D. (X±K)= SD (X)
- Standard deviation is not independent of charge of scale. In other words, if all values of a variable X are multiplied or divided by some constant “K” the standard deviation changes accordingly. Mathematically,
SD= (KX)= K SD (X)
SD= (X/K)= (1/K)SD X
- If X is a variable and K1 and K2 are constants, SD (K1X±K2)= K1 SD (X)
- Standard deviation has some units as that of a variable.
- Standard deviation has following relationship with the mean deviation and quartile deviation.
4SD=5MD=6QD
- If arithmetic mean and standard deviation of two or more sectors are given, combined standard deviation can be calculated by using the following methods:
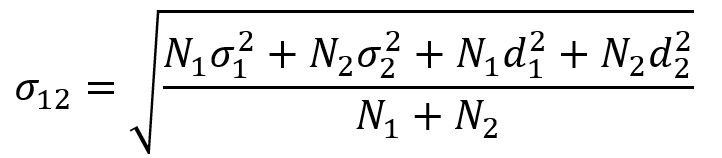
- Standard deviation is always positive.
- Standard deviation is always calculated by taking deviations from Arithmetic Mean. Therefore, sum of squares of deviations from Assumed Mean is minimum.
- In case of symmetrical distribution or Normal Distribution, following area relationship holds good:
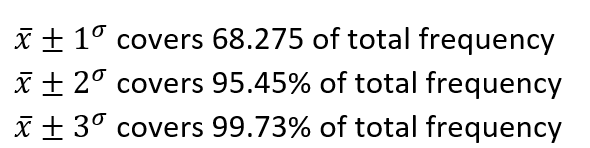
MERITS OF STANDARD DEVIATION
Standard deviation is the most important and extensively applied measure of dispersion. This measure of dispersion satisfies all the essentials of an ideal measure of dispersion.
1. When samples are combined in different ways, the standard deviation of the combined data can be calculated from means and standard deviations of the original data.
2. In the normal distribution, standard deviation plays a pivotal role.
3. The sum of squares of the deviations from the actual mean, is the minimum. Thus, standard deviation is the best measure of dispersion
4. Standard deviation is liable to further algebraic treatment.
5. The standard errors of various methods are based on their standard deviations.
DEMERITS OF STANDARD DEVIATION
1. Standard deviation does not give any idea about the exactness of the measurement. The standard deviation can be higher in a particular period as compared to earlier period. It is just possible that there may be higher value of standard deviation due to which there is greater variability in the distribution. But it may be due to the reason that in the first year, the mean may be low than in the second year. This result can be true if the two means are uniform. Hence it is clear that standard deviation depends on arithmetic mean and so it is likely to change with the change in the size of mean.
2. Standard deviation is referred to only as an absolute measure of dispersion and thus it cannot be used for comparing the two phenomena.
3. The extreme items gain great importance. Those items which are close to mean, do not gain much importance. It is due to this reason that the of big deviations would be proportionately higher than the square of small deviations.
4. Standard deviation is more difficult to be measured as compared to other measures of dispersion because square root is involved.
VARIANCE
Variance is square of Standard Deviation. The term was first used by RA. Fisher in 1913. The measure of variation is liable for further quantitative analysis. If we are dealing with a phenomenon affected by a number of variables, in that case variance helps us in separating the effects of different factors.
Smaller the values of , the lesser the variability or greater the consistency and vice- versa:
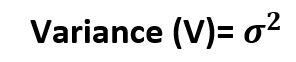